The Roadblocks to Human-Level AI Problem Solving
- Workplace
- Feb 13
- 2 min read
Updated: Feb 16
Developing AI that can truly emulate human expert problem-solving remains a significant challenge. Several key obstacles hinder progress:
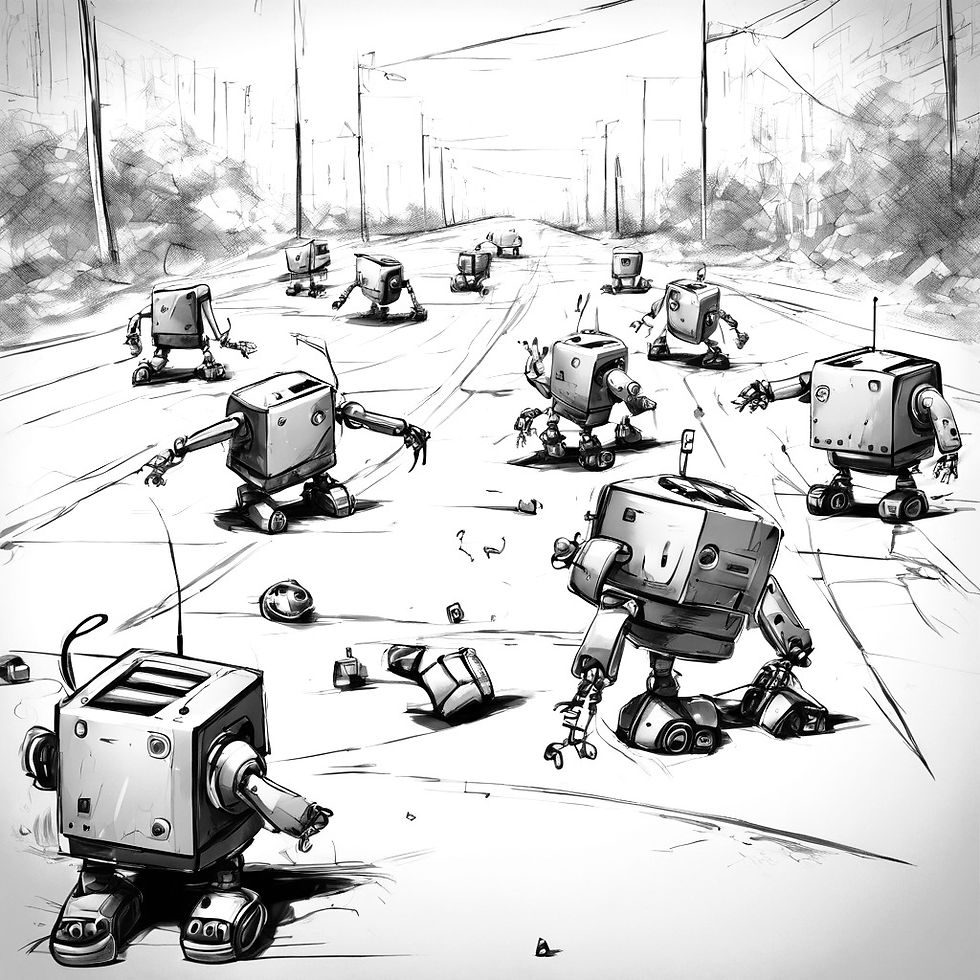
Data Limitations:
Quantity and Quality: Real-world data is often insufficient, noisy, and biased. The "10x rule" (requiring at least 10 times more data than features) is a lower bound; much larger datasets are typically necessary for a machine learning model to effectively learn and generalize.
Machine Bias: Concerns around the data used are paramount. If the training data reflects existing human biases, the resulting machine learning model will likely perpetuate and even amplify these biases, leading to unfair or discriminatory outcomes. Addressing and mitigating these biases is crucial for the ethical and responsible development of AI systems.
Implementation Hurdles:
Aligning with Business Goals: Successfully integrating AI requires clear alignment with business objectives.
Resource Constraints: Securing necessary infrastructure, resources, and skilled personnel can be a major hurdle.
Stakeholder Engagement: Effective communication and collaboration with stakeholders are crucial for successful AI adoption.
Transparency and Trust:
Lack of Explainability: Many AI systems are "black boxes," making it difficult to understand their decision-making processes. This hinders trust and wider acceptance.
Explainable AI (XAI) techniques and human-in-the-loop design are essential to improve transparency and build trust.
Key Considerations for Success:
Data Excellence: Go beyond the "10x rule" to address the complexities of real-world data.
Advanced Model Development: Explore meta-models and pathfinding techniques to guide AI development and improve problem-solving efficiency.
Human-AI Collaboration: Leverage the strengths of both humans and AI through collaborative approaches. This fosters greater accuracy, explainability, and adaptability.
By addressing these challenges and embracing innovative approaches, we can move closer to AI systems that truly partner with humans to solve complex problems effectively.